spent christmas weekend making this demo of a robot picking up cubes (it doesn’t know which color cube is where beforehand)
Wellll okay this could be a long post but I’m pretty sleep so I’ll just “liveblog” it and not worry too much about making it a tutorial / step-by-step instructions / details. (it’s a two day hackathon project anyway)
two out of three of my roommates are also roboticists, so we (the three of us) started on a house project: a robot arm.
one of us want to focus on designing the arm from scratch (started on cable driven, now focused on cycloidal gearboxes) , and two of us wanted to get started on the software side (divide and conquer). so we got a commercial robot arm for $200: the lewansoul 6dof xarm.
i decided over christmas that it was time to give up on work and do side projects. so i spent two days and hacked together a bare minimum demo of a “helper robot.” we eventually want something similar to dum-E robot in iron man, a robot that can hand you tools etc.
the minimum demo turned out to be verbally asking the robot to pick up a cube of a specific color
the name
i felt mean to call a defenseless robot “hey dummy” all the time, so went with suggestion to use “hey smarty” instead …
probably the dum-e reference will be lost on everyone, since I only remembered jarvis in iron man before starting this project
first print some cubes
T^T the inevitable thing that happens when you get a 3d printer, you crush your meche soul and print cubes. then cover in tape to get different colors
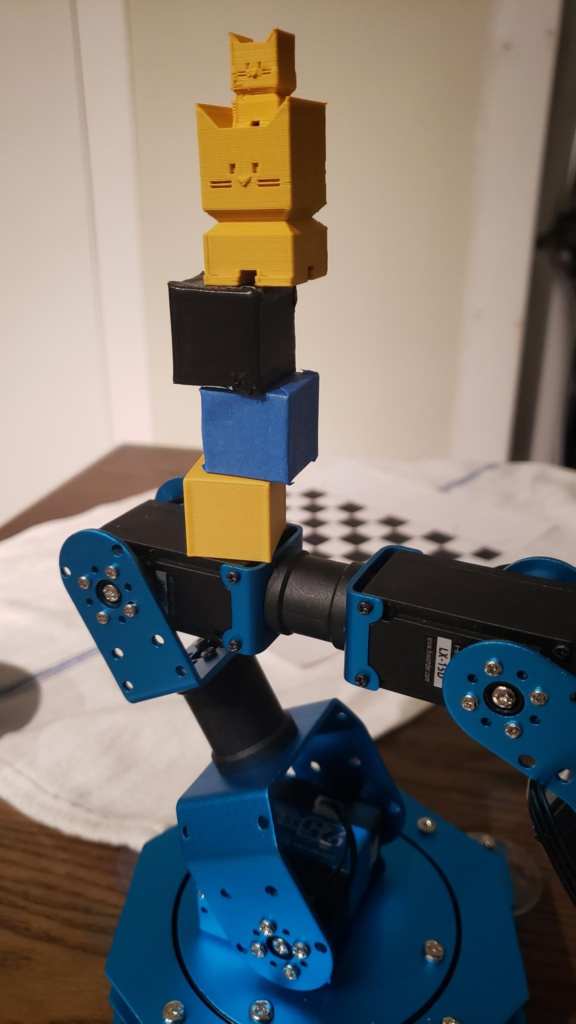
lewansoul robot arm python library
there are several python libraries written, we worked with https://github.com/dmklee/nuro-arm/
which implements already the IK (inverse kinematics) so that we can give x,y,z coordinates instead of joint angles for each of the six joints. runs smoothly (after fixing a udev permissions issue). the library’s calibration required us to move the servo horn a little from how we initially put the robot arm together.
quickstart
$ git clone https://github.com/dmklee/nuro-arm/
$ cd nuro-arm
$ ipython
>>>
from nuro_arm.robot.robot_arm import RobotArm
import numpy as np
robot = RobotArm();
robot.passive_mode() ;
# now robot servos do not fight you. physically move robot arm to desired pose
>>>
test_pose_xyz = np.round(robot.get_hand_pose()[0], decimals=4);
print('---> ', test_pose_xyz, ' <---')
>>>
POS_MID = [0.200, -0.010]
Z_SUPER_HI = 0.10
OPEN = 0.5
robot = RobotArm()
xyz = np.append(POS_MID, Z_SUPER_HI)
robot.move_hand_to(xyz)
robot.set_gripper_state(OPEN)
additional things to try: provides GUIs
python -m nuro_arm.robot.record_movements
python -m nuro_arm.robot.move_arm_with_gui
NOTES if have difficulty seeing robot on ubuntu, re: udev, on 20.04
sudo vi /usr/lib/udev/rules.d/99-xarm.rules
SUBSYSTEM=="hidraw", ATTRS{product}=="LOBOT", GROUP="dialout", MODE="0666"
sudo usermod -a -G dialout $USER
sudo udevadm control --reload-rules && sudo udevadm trigger
pip install easyhid
sudo apt-get install libhidapi-hidraw0 libhidapi-libusb0 # maybe needed?
(on 18.04 it is /etc/udev/rules.d)
troubleshooting:
import easyhid
en = easyhid.Enumeration()
devices = en.find(vid=1155, pid=22352)
print([dev.description() for dev in devices])
# should something like
# devices ['HIDDevice:\n /dev/hidraw2 | 483:5750 | MyUSB_HID | LOBOT | 496D626C3331\n release_number: 513\n usage_page: 26740\n usage: 8293\n interface_number: 0']
make sure robot is plugged into laptop
voice interaction
the other part of the demo is voice interaction. two parts:
speech to text: recognize the trigger phrase and the commands after. this is modelled after the amazon alexa, apple siri, google home style of voice interaction with “AI”, where you say a trigger phrase.
now based on our inspiration it would be “hey dum-e” but this felt pretty mean, so was suggested to use “hey smart-e” instead 🙂
voice interaction part A: generating smart-E’s “voice”
from an earlier project (creating a welcome announcer in the lobby for the gather.town for ICRA 2020) I knew I could use mozilla TTS to generate nice-sounding voice from text.
https://github.com/mozilla/TTS
(as opposed to the default “espeak” which sounds like horrible electronic garble)
was rather annoying install at the time (a year ago?) but seems to be a cleaner install this time around, however!! all the instructions are for training your own model, vs I just wanted to use it to generate audio.
So here’s a quickstart to using Mozilla TTS.
~$ python3 -m venv env && source ./env/bin/activate && which python && pip3 install --upgrade pip && pip3 install wheel && echo done
~$ pip install tts
~$ MODEL="tts_models/en/ljspeech/glow-tts"
~$ tts --text "What would you like me to do?" --model_name $MODEL
~$ tts --text "What would you like me to do?" --out_path "./whatwouldyoulikemetodo.wav"
> tts_models/en/ljspeech/glow-tts is already downloaded.
> Downloading model to /home/nrw/.local/share/tts/vocoder_models--en--ljspeech--multiband-melgan
> Using model: glow_tts
> Vocoder Model: multiband_melgan
> Generator Model: multiband_melgan_generator
> Discriminator Model: melgan_multiscale_discriminator
> Text: What would you like me to do?
> Text splitted to sentences.
['What would you like me to do?']
> Processing time: 0.7528579235076904
> Real-time factor: 0.3542274926029484
> Saving output to whatwouldyoulikemetodo.wav
$ play tts_output.wav
voice interaction part B: giving smart-E “intelligence”
hot stars, in the last year or two we gained a realllllly nice speech2text library. used to be that if you wanted reasonable real-time-ish text transcription, you needed to get a google API key and use google API.
now you can just pip install the Vosk library, download a 50 mb file, and get real-time offline transcription that actually works! and if you want Chinese transcription instead of English, just download another 50 mb model file.
https://alphacephei.com/vosk/install
i’m liking this future 🙂
it just works! no training required for different voices interesting correction going on (probably language model to find likely phrases?)
quickstart
$ git clone https://github.com/alphacep/vosk-api
$ cd vosk-api/python/example
$ wget https://alphacephei.com/kaldi/models/vosk-model-small-en-us-0.15.zip
$ unzip vosk-model-small-en-us-0.15.zip
$ mv vosk-model-small-en-us-0.15 model
$ pip install sounddevice
$ python test_microphone.py
now to make the keyword recognition happen.
def find_keyword(voice_result):
keywords = ['marty', 'smarty', 'smart']
(In a while True loop -- see test_microphone.py in the vosk repo)
if rec.AcceptWaveform(data):
sentence = rec.Result()
if not state_triggered:
found_keyword = find_keyword(sentence)
if found_keyword:
state_triggered = True
# if state_triggered, then move on to listening for colors
arucotags in python
how to locate the cubes? okay, actually i simplified this step in interest of finishing something in a weekend (before leaving for home for the holidays), and only had three positions. instead the issue was finding which color cube was where.
how to distinguish yellow cube from yellow stickynote? or black cube from checkerboard? i solved it by putting tags on the cubes and sampling (in 2d space) the color slightly above the tags to determine cube color.
I made a quickstart here for working with arucotags in python: https://gist.github.com/nouyang/c08202f97c607e2e6b7728577ffcb47f
note that the gist shows how to get 3d out, vs for the hackdemo i only used the 2d.
what color is the cube
this was actually annoying. in the end i assume there are only three colors and do a hardcoded HSV filter. Specifically, use hue to separate out the yellow, and then value to separate black from blue. (this is hack – note that the logic is flawed, in that black can be any hue)
crop = image[y-rad:y+rad, x-rad:x+rad]
color_sample = crop.mean(axis=0).mean(axis=0)
rgb = color_sample[::-1] # reverse, color is in BGR from opencv
rgb_pct = rgb / 255
hsv_pct = colorsys.rgb_to_hsv(*rgb_pct) # fxn takes 3 values, so unpack list with *
hsv = np.array(hsv_pct) * 255
print('rgb', rgb, 'hsv', hsv)
hue = hsv[0]
val = hsv[2]
closest_color = None
if 10 < hue < 40:
closest_color = 'yellow'
if hue >= 50:
if val > 90:
closest_color = 'blue'
else:
closest_color = 'black'
additional notes
i’ll think about making a public snapshot of the git repo for the demo, perhaps. for the above code samples, my file directory looks like so:
$ ls
env
nuro_arm
speech_output # wav files from mozilla tts
voice_model
hackdemo.py
$ python hackdemo.py
Note
The pop culture reference for smart-E
this is the voice interaction, 38s sec to 62 sec
for longer context of the dum-e scenes, see
creepy voice output
it’s a deep learning model, so it gives unpredictably different results for hey! vs hey!! vs hey!!! and seems to do poorly on short phrases in general. (also you can’t force any specific intonations, like a cheerful “hi there!” vs a sullen “hi there”)
here’s the creepy output of the default model with just “hey”
funny bugs
i got the voice recognition to recognize the colors, but it seemed like the program would get caught in a loop where it would reparse the same audio again and again. i spent a while going “??? how do python queues work, do they not remove items with get()???” and clearing queues and such. eventually my roommie pointed out that, i have it give the response “okay picking up the yellow cube” so it was literally reacting to its own voice in an infinite loop 0:
it was fun to think about the intricacies of all the voice assistants we have nowadays
next steps
on the meche side, build an actual arm that can e.g. lift a drill (or at least a drill battery)
on the cs side, we will continue working with mini arm. bought a set of toy miniature tools (used for kids). we’ll use probably reinforcement learning to learn 1. what pixels correspond to a tool 2. which tool is it 3. where to grasp on the tool. and then 4. how to add new tools to workspace
decisions: match cad models? or pixels? to determine which tool
on the voice side we also have to do some thought about how people will interact with it. “hand me the quarter-inch allen wrench” or “hex wrench” or “hex key” or “t-handle hex” or “ball-end hex” etc., asking for clarification, …
currently we just figured out how to go from webcam image (x,y pixels) to robot frame (x,y,z millimeters) so you can click on the camera image and the robot will move to that point in real life